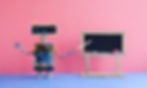
Today, black box AI algorithms are pervasive. As consumers and web users, our online experiences are often defined by algorithms that we neither control nor understand.
In the word of HR technology, hiring algorithms are all-to-frequently equally opaque. They are most commonly used in two distinct ways: To identify “passive” job seekers by analyzing data like social media profiles and resumes previously uploaded to job boards, and to identify best-fitting “active” job candidates by analyzing content in resumes and other application materials. While they are widely used and relied upon, their logic that can be difficult to explain – even by those who design and engineer them.
Several recent high-profile incidents involving AI hiring algorithms have exposed the flaws inherent to black box systems. As a result, industry groups and regulators have begun introducing legislative bills intended to minimize the discriminatory effects that are inherent to opaque algorithmic hiring technologies.
Unintended consequences of looking backward
Back in 2014, a team of engineers at one of the world’s largest technology companies began building an experimental candidate evaluation solution that would review the language in job applicants’ resumes with the objective of automating the search for top talent. The hiring algorithm graded each applicant’s suitability on a 5-point scale. By 2018, the experimental evaluation solution was shelved. The company had discovered its 5-point hiring solution had inadvertently discriminated against women, and the company quickly shut it down.
So, what happened?
Training data typically takes the form of a very large labelled or unlabelled dataset that is used to teach a machine learning model how to identify features that are relevant to a business objective. In the above example, engineers trained the model on language contained in all the resumes that candidates had submitted to the company over the prior ten-year period. Over that period however, the company’s workforce was predominantly male, and the algorithm recognized the overweighting of language associated with resumes belonging to males and assumed that male gendered candidates were preferable. The success or failure of this form of “lookalike” analysis, which measures similarity between past and prospective hires based on features in an ML model, depends on the quality of the training data and also the degree to which historical data represents the future objectives of the company.
Explainability matters
Why do black box algorithms create concern for HR practitioners? FICO’s 2021 State of Responsible AI found that 65% of companies can’t explain how specific AI model decisions or predictions are made. “Explainable” is a term used to characterize the inner workings of an AI model such that its impact, its potential biases, and its route to outcomes can be understood. Explainability is crucial for any organization to have trust and confidence in the AI models it employs, and to be able to hold itself accountable for its AI-assisted decisions.
From the vantage point of human-centered research areas such as computational social science and social psychology, interpretable models often move science forward more than do black box models. Finding concrete characteristics that predict job performance is more useful for both theory and in practice than discovering that one black box model outperforms another by a few percentage points (see Rudin, 2021).
Appropriate data is critical
More recently, several technology companies have attempted to develop AI hiring algorithms that assess a candidate’s personality based on the language data contained in their LinkedIn profile. These technologies then compare each applicant’s personality to that of an “ideal candidate” to identify top candidates within the applicant pool (Rhea et al., 2022). However, the language data source that these solutions use presents a significant problem. Simply put, the language contained in resumes and LinkedIn profiles isn’t suitable for language-based personality analysis.
What makes some language sources suitable while others are not? At the foundation of language-based personality assessment is the requirement for “natural language”. While the distinction between natural language and formal structured language may seem slight, the psychological differences are substantial: Formal language is carefully crafted language like that which is found in prepared speeches, LinkedIn profiles, and resumes. Natural language, however, is conversational language – the everyday language we use to communicate with others, answer questions, debate, and discuss.
While formal language may be suitable for traditional NLP methods like topic extraction, natural language contains the nuances, double meanings, and ambiguity that reflects the humanity of our language. It is this natural language that can be analyzed to understand the unique differences among individuals. Solutions that attempt to infer personality based on data sources containing exclusively formal language, therefore, are inherently flawed.
The function of natural language
While AI-based algorithmic decision-making systems garner considerable attention and investment, in the realm of candidate evaluation there are alternative algorithmic approaches that are validated and more suited to the task at hand. Unlike black box algorithms, these approaches are also explainable, which makes them well suited to the needs of HR practitioners, and positions them to satisfy the requirements of industry regulators.
Rather than the black box approach of treating language data as features in a model, the alternative is to interpret the language data from the perspective of a psychologist – recognizing that the unique ways that people use language is a direct reflection on their uniqueness as an individual. Decades of research has been conducted to understand how people communicate, to understand not only the content of what they are saying, but also to understand the unconscious choices people make when they assemble words to form sentences, and what these choices imply about the person, their personality, aspects of their psychology, and the spectrum of feelings they are experiencing in the moment.
When people communicate, they use two different categories of words – “content words” and “function words”. Content words are the nouns, verbs, adjectives, and adverbs people use to articulate the specifics of what they are talking about. Function words are primarily pronouns, prepositions, and conjunctions -- words that exist to explain or create structural relationships into which the content words fit. From a physiological perspective, function words are also unique because they are processed differently in the brain -- unlike content words they are processed largely unconsciously.
While these details may seem insignificant, experts in psychology and linguistics have long recognized just how significant and predictive people’s use of function words can be. In thousands of peer-reviewed studies over the past 20 years, researchers have proven the predictive value of function words, and the important role they play in understanding human psychology, personality, mental wellness, emotions, interpersonal interactions and group dynamics.
In his capacity as Chair of the Psychology Department at the University of Texas, Dr. James W. Pennebaker, was a pioneer who recognized the outsized power and importance of function words in understanding human psychology. Dr. Pennebaker’s research and approach is extremely well documented and extensively validated. His underlying approach involves using teams of experts in language and psychology to categorize words and phrases into categories the reflect the psychological processes that are involved in using them. For example, words that people use that relate to the psychological concept of certainty include words like “absolutely, commit, and definite”. Hundreds of such categories exist and are comprised of over 19,000 words, and each category reflects a different psychological phenomenon. Psychology, personality, mood, and other factors lead different people to unconsciously select different words even when they are saying the same thing. For example, people who are extroverted will typically use higher frequencies of words that relate to social processes and assertiveness than people who are introverted. (Ireland & Mehl, 2014; Mehl, 2006).
An explainable approach to algorithmic hiring
It is upon this foundation that a large body of research has established how people’s everyday natural language use in writing and spoken conversation reliably reflects personality (Chung & Pennebaker, 2007). For example, people who use more first-person singular pronouns tend to score higher on neuroticism than those who use fewer self-focused pronouns, very much characteristic of the negative self-focused facets of neuroticism (e.g., anxiety, depression, self-consciousness; Tackman et al., 2019). The language categorization approach that's core to language psychology can be used to identify candidates with specific personality attributes that prior research, hiring managers and HR deem to be most important to success in a particular role.
Language psychology is what underpins the Receptiviti platform, and it is uniquely suited for building candidate evaluation solutions for several important reasons, the most important being its inherent explainability: The extensively validated body of research that associates personality characteristics with the unique ways that each individual uses language provides a foundation for evaluating candidates that is not reliant on potentially biased historical hiring data. The approach’s explainability comes from the broadly available public research into the relationship between language use and personality characteristics that has been conducted by Dr. Pennebaker and hundreds of social-personality psychologists and computational social scientists.
It's a different and deeply human way of thinking
Integrating technology that uses language psychology methods in personnel selection offers employers an objective and explainable way of understanding what makes each job candidate unique and how they might behave in a particular workplace setting.
While black box AI algorithms will continue to proliferate, regulators will increasingly push for legislation to address problematic implementations. The long-term viability of AI, and the viability of hiring algorithms specifically, will require a shift in the way we think about progress.
The growing proliferation of AI is unquestionably exciting, and while the adoption of AI models can accelerate outcomes in certain domains, they can also present significant challenges in others. Hiring technology is one of these problematic areas, and as regulators become more skeptical of AI-based algorithms, methods that employ language psychology present a powerful alternative for solving the challenge.