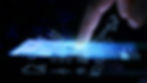
In this study, the researchers aimed to develop a screening tool for depression that can be easily administered by analyzing the patient’s tweets. Depression is a common mental health disorder, and early detection can lead to better treatment outcomes. With the correlation between frequent social media use and depression, the researchers proposed a novel screening tool system that incorporates an artificial neural network (ANN) to analyze the patient’s tweets. The researchers used a dataset curated from Kaggle, and four algorithms were used to train the model. The SVM model achieved the best performance, but the Perceptron model was chosen due to its faster speed.
The results showed that the proposed screening tool has a high accuracy of 98%, and it is a promising approach for screening depression. The use of social media data for screening tools can provide a less invasive and more efficient approach for identifying individuals at risk of depression. The study highlights the potential of utilizing AI and social media data for developing more effective screening tools for mental health disorders. A novel screening tool that utilizes social media data can help improve the early detection and treatment of depression.